DATA EXCELLENCE: FOR SMART PRODUCTION
THE USE OF THE COMPANY‘S INTERNAL DATA RESOURCES AND THE CONFIDENT HANDLING OF THE MOST IMPORTANT DATA-DRIVEN TECHNOLOGIES ARE THE BACKBONE OF INDUSTRIAL DIGITALISATION.
They are the prerequisite for improving operational excellence, agility and planning accuracy, reducing costs and risks and gaining transparency over complex supply chain networks. based on study results, current applied research and project experience, five topics can be defined that are highly relevant for the digital transformation in industry and form the focus of the ROI analytics lab.
BUSINESS INTELLIGENCE & PROCESS MINING
Business Intelligence (BI) and Process Mining are particularly effective tools for gaining a comprehensive insight into actual process events and sustainably increasing efficiency - for example by discovering process steps and interfaces where time losses or irregularities occur. For this purpose, log data from IT systems (ERP, CRM, MES, CAQ, ...), but also from other structured and unstructured data sources, are first extracted, integrated and prepared for data analysis. The prerequisite for this is that processes leave a digital trace, for example through time stamps, IDs or documented activities. Based on this, actual process flows are reconstructed and visualised. This creates an objective and complete picture that serves as an optimal starting point for process adjustments. At the same time, this is the basis for automating process management (e.g. through automated report generation and distribution or KPI-dependent alerts). Particular added value is created in cross-departmental and cross-functional processes.
PREDICTIVE ANALYTICS
The correlation of different parameters and their influence on processes and quality repeatedly proves to be a black box in production. As a result, important tools for reducing losses and achieving sustainable improvements are missing. With the predictive analytics approach, all relevant parameters can be uncovered, their correlations analysed and integrated into a dynamic, learning data model. This creates the basis for identifying process-related weak points, reducing maintenance and quality costs, improving decision-making quality and controlling production in real time with foresight. This requires a circular approach in which business model analysis, data preparation (understanding, integration, preparation), data modelling, evaluation, implementation and ongoing adaptation are intertwined. Typically, the successful development of a predictive analytics solution requires the cooperation of several experts. These include in particular process experts from the departments concerned, data scientists and data engineers as well as the IT department.
MODEL PREDICTIVE PROCESS CONTROL
Model Predictive Process Control (MPC) is an intelligent and lean method for optimising processes in real time on the basis of dynamic models, especially for the process industry. Based on a real-time-capable IT infrastructure, the focus is on the next production sequence in each case, while at the same time the overall process is taken into account. MPC allows effective, predictive control even without setting up significantly more complex digital process twins. The method is particularly helpful in accelerating the start-up of production processes, mastering new technologies and processes, and reducing losses and waste.
SIMULATION & OPTIMIZATION OF PROCESSES
Simulation studies for complex or cost-intensive processes help to make better decisions and to estimate the long-term effects of the process design. The approach is based on the construction of alternative scenarios that take into account dynamic influencing factors such as seasonality or disruptions in the value chain. For example, investment decisions, dimensioning of buffer stocks and logistical capacities or value flows can be simulated. The decisive factor here is a continuous, methodically supported simulation process that ranges from the definition of goals and problems to the design and validation of the simulation model and the collection of data to the visualisation of results. The availability and validity of the data used and the choice of the right methodology for building the simulation model prove to be critical factors. In addition, only the combination of a powerful but lean software solution with comprehensive technical and process expertise guarantees that the objective is precisely formulated and the results are correctly interpreted.
ROBOTIC PROCESS AUTOMATION (RPA)
RPA solutions automate processes by imitating human activities. In doing so, they directly access systems such as ERP, CAD or even mail programmes. Interesting fields of application for RPA arise where process automation with a comprehensive solution does not pay off. In production, this is particularly the case in indirect areas, such as reporting. For example, in the context of shop floor management, key figures from different sources, such as Excel spreadsheets or ERP and production systems, have to be merged. Tasks like these are typically error-prone and resource- and time-intensive. In such contexts, RPA solutions help to reduce headcount and costs, improve the quality and auditability of workflows and achieve high process scalability. Today, there are numerous powerful and userfriendly tools for the implementation of RPA projects that do not require excessive IT expertise. Rather, success depends on effective interaction between process analysis, process design and software, as well as effective interdepartmental collaboration.
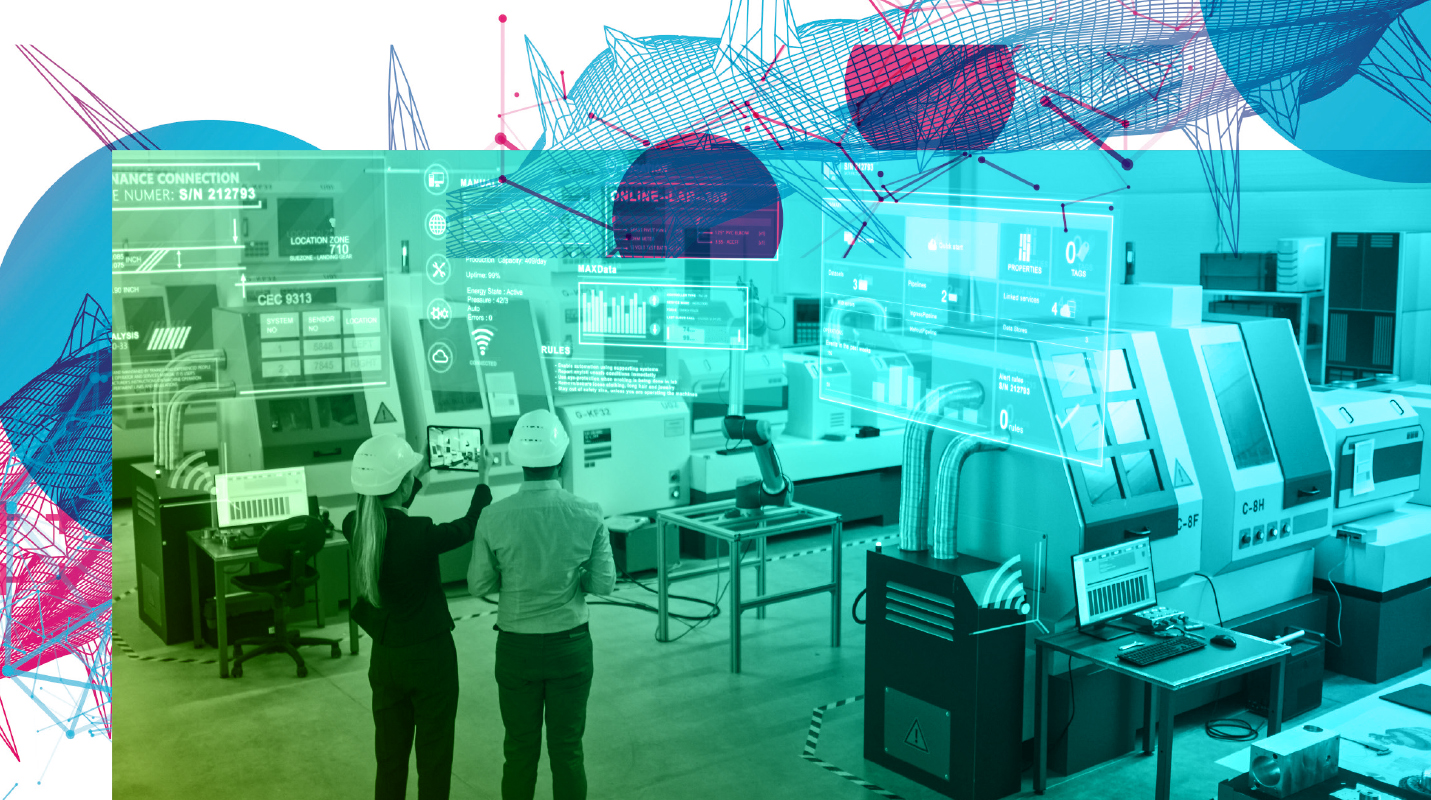