TOPICS & NEWS
Articles and interviews on current trends, technology and industry challenges, information on our consulting services, seminars and events as well as company topics:
Here you can find out what drives EFESO.
The technology of digital transformation is increasingly becoming a commodity
Professor Bick, many companies today use data to improve and develop customer services and products. But what does this look like in a smart factory?
Most companies today use data in the areas you just mentioned. What many have not yet tapped, however, is the wealth of data in areas ranging from the delivery of primary materials to the delivery of the finished product, including intralogistics, distribution, external logistics, etc., i.e. within the manufacturing company. Companies are increasingly starting to tackle this issue. How do I use information in a concrete way, how do I make the topic of data tangible for myself in order to generate added value for my company? I'm not just referring to our own production, but the entire value chain.
Why are we not more advanced in the use of data in production?
The subject is still far from being an industry standard. There are still many companies that do not follow a clear line when it comes to data acquisition in production or its use. And there are also specific challenges. For example, large companies have the necessary resources but sometimes fail because of their complexity. Smaller companies, on the other hand, often lack the clout needed to take decisive action.
Do you, therefore, need different approaches for large and small companies?
The size of the company is only one aspect. What matters is the attitude and cultural perspective with which you approach the topic. What freedoms do you allow for and do you factor in failure? Where must clear and transparent guidelines be set? Are you prepared to start small but fast and proceed step by step, or do you spend six months discussing the right IT infrastructure? How do you deal with limited resources and how much do you challenge existing frameworks? The answers will always be different depending on the structure of the company – but the questions must be asked by everyone who sets out on the path to creating a smart factory.
How do I, as a company, specifically proceed in order to get started with data usage?
First, you need a team that has a high level of intrinsic motivation. As management, you can only initiate such a transformation top-down to a very limited extent. This not only applies to corporations, but also to medium-sized companies. And you have to have a more concrete idea of where you want to go, a vision for the future. And this vision must be supported by a strong commitment from management. And then you have to let the teams work in peace to create a bottom-up movement. In an agile working method, progress must be reviewed in short cycles so that you can keep an eye on where you want to go and not get bogged down. That is the framework.
Specifically, you should start such a project with a point of view analysis in order to compare your own possibilities and potentials with the benchmark solutions already implemented. And on the basis of this, perhaps two or three initiatives should be defined to start with. After all, this is also a mistake that happens very frequently: You want to set off an avalanche straight away, fire off fireworks on various fronts. I haven't heard of any cases where this approach has been effective.
What role does technology play in this process, how heavily do the initiatives in the smart factory depend on it?
In my opinion, the importance of technology for building the smart factory is greatly overestimated. Today, there are very good and inexpensive standard solutions and web services that can be accessed quickly. The technology of digital transformation is increasingly becoming a commodity. This even applies to artificial intelligence, where there are an increasing number of solutions that can be used very effectively without in-depth expertise. Differentiation is, therefore, more likely to be achieved by having a clear, well-founded and creative plan and from this deducing which tool is best suited to the given task and creates the greatest added value, and which information and which data model is needed.
What should you watch out for when constructing the necessary data model?
The decisive factor is consistency, so that the requirements of all areas are covered uniformly, and the process is not hindered by the proverbial silos. In addition, there must be a high degree of flexibility so that the model grows with it and can successively display additional information. It is less a question of the technological basis than of the organisation. And then a system landscape that is as transparent as possible should be set up with existing standard solutions. It is important to pay attention to the relationship between requirements and complexity in order to achieve a good cost-benefit ratio: it is better to have a system that perhaps only meets 80 percent of my requirements, but has a 40 or 50 percent lower degree of complexity. For the remaining 20 percent, you can usually find pragmatic solutions.
When starting to use data, should the focus be on a specific process or a specific area of production?
Both are conceivable. For example, you can look at the quality assurance process and ask yourself the question of how errors can be quickly detected, analysed and remedied through intelligent data use throughout the entire production chain. The other option is to optimise a single area, such as a model line. This can be a spatially delimited area or a business process that can be easily influenced. So it's all about the target image on the one hand and a quick start on the other – where you have the optimal conditions to create added value with data. Whether it is horizontal or vertical is not important.
What is the nature of this added value created by intelligent data use in the factory?
On the one hand, it's about efficiency and costs. For example, when anomalies and patterns are recognised early, and system malfunctions or downtimes are avoided. This has a direct and significant effect on the OEE and therefore also on the costs. This is the deployment scenario for predictive and prescriptive maintenance.
Another enormously important aspect is transparency and precision, for example, in planning processes and controlling throughput and delivery times. You can use data from a production process to determine fluctuations, errors or process instabilities and use this data to make improvements. And bull whip effects can be avoided by ensuring that the resources in the supply chain are optimally coordinated across companies. Transparency also means that you are always able to provide customers with information about the production process. So far, this is anything but a matter of course.
And finally, as I already mentioned, it's about quality. You can use data to carry out quality controls even more quickly, to find the causes of errors at an early stage and to eliminate them permanently. When it comes to quality, speed is therefore right at the top of the agenda. This can be supported in an extremely smart way by using simple tools such as a stored error plan for error recording. Using a data-driven approach, it is possible to initiate such correction loops. In the area of error root cause analysis and error evaluation, you can then talk about the use of AI.
In view of this potential, it is surprising that the development around the topics Smart Factory and Industry 4.0 is not picking up speed.
I do not take such a critical view of this. Every radical innovation needs a certain start-up period. This initial phase is now behind us. In the meantime, many technological hurdles have been overcome, standards have been created, and there are numerous reliable use cases and experiences. This is impressively demonstrated, for example, by the winners of the Industry 4.0 Awards presented annually by ROI. An increasing number of companies have also approached the topic culturally and created the necessary structural conditions. And last but not least, industrial digitisation has impressively demonstrated its potential when faced with the extreme conditions of the coronavirus pandemic. I am therefore very optimistic that we will experience an enormous momentum of digitisation in production over the coming years.
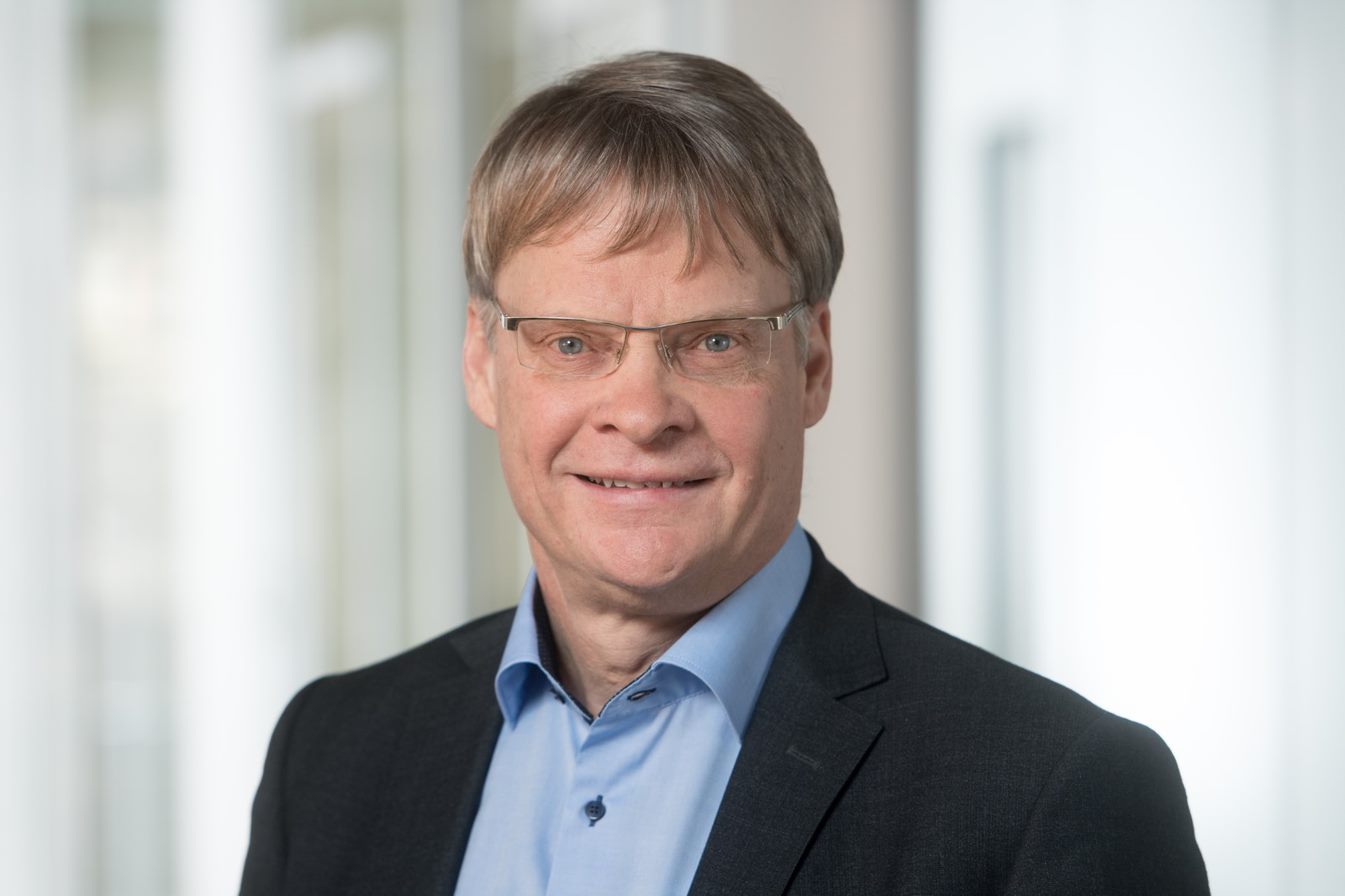
PROF. DR.-ING. WERNER BICK
Senior Partner
Prof. Dr.-Ing. Werner Bick teaches at the 'Technische Hochschule Regensburg' and serves as Senior Partner of ROI Management Consulting AG in Munich since 1999.
Prof. Bick brings his expertise to bear on improving internal and cross-company logistics, optimizing production, and increasing efficiency and effectiveness in development and the product development process.
Prior to joining ROI, Prof. Bick worked for Knorr-Bremse AG as head of logistics and head of the production segment.
After completing his studies in mechanical engineering, Prof. Bick first worked for Milberg GmbH as a consultant for assembly and structure design. During this time, he completed his dissertation on the technical and economic optimization of assembly systems.